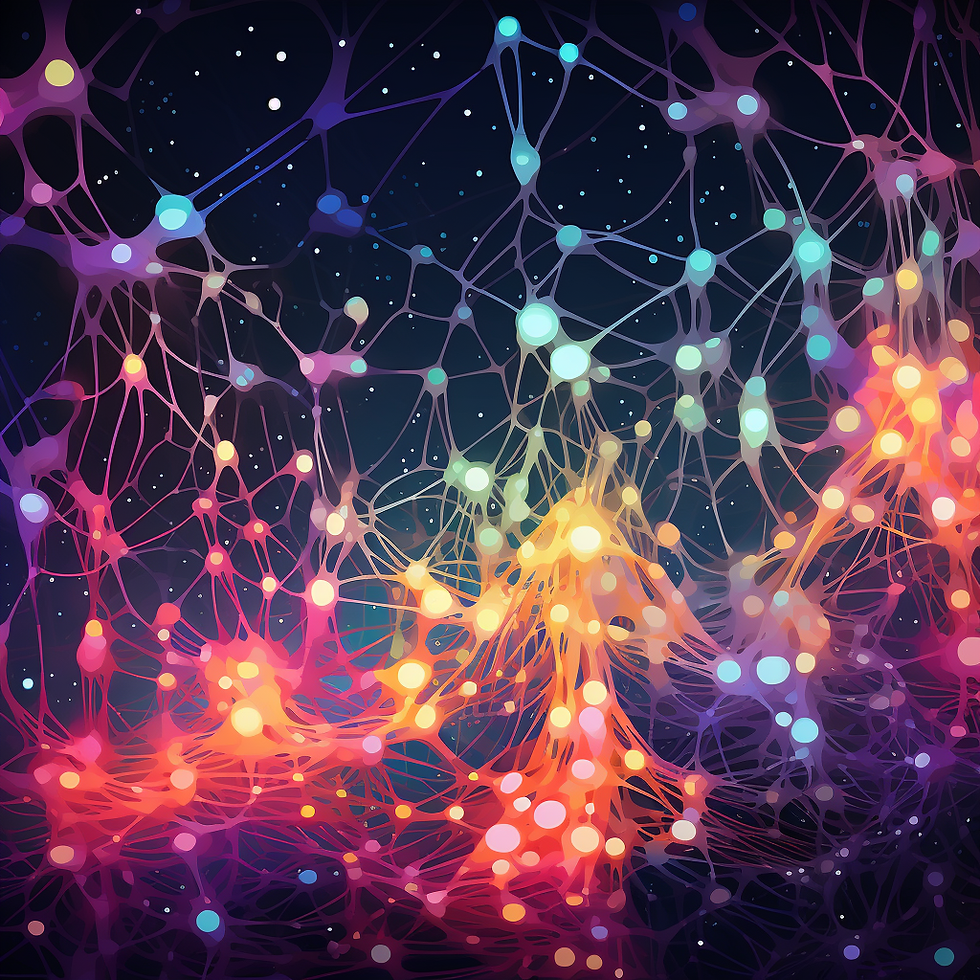
Introduction
In the enchanting realm of Artificial Intelligence (AI), neural networks stand as a cornerstone, mimicking the intricate workings of the human brain. This blog aims to demystify the complexities of neural networks, providing a beginner-friendly exploration of this fundamental concept that powers many AI applications.
The Basics of AI Neural Networks
At its essence, a neural network is a computerized model inspired by the structure and function of the human brain. Comprising interconnected nodes, or artificial neurons, these networks process information in layers, with each layer contributing to the extraction of patterns and features from input data.
How Neural Networks Learn
Neural networks learn through a process known as training. During this phase, the network is exposed to a vast dataset, and its parameters are adjusted iteratively to minimize the difference between predicted and actual outcomes. This learning process allows the network to generalize and make accurate predictions on new, unseen data.
Layers of Understanding: Input, Hidden, Output
Neural networks consist of layers, each serving a unique purpose. The input layer receives data, the hidden layers extract features, and the output layer produces the final result or prediction. The depth and complexity of these layers contribute to the network's ability to understand and process information.
Types of Neural Networks
There are various types of neural networks, each tailored for specific tasks. Convolutional Neural Networks (CNNs) excel in image recognition, Recurrent Neural Networks (RNNs) are adept at processing sequential data, and Generative Adversarial Networks (GANs) can create realistic content. Understanding the application of each type is crucial for leveraging neural networks effectively.
Real-world Applications
Neural networks power an array of real-world applications, influencing our daily lives. From voice assistants understanding natural language to facial recognition unlocking smartphones, the versatility of neural networks extends across industries, including healthcare, finance, and autonomous technologies.
Challenges and Future Frontiers
While neural networks have revolutionized AI, they are not without challenges. Issues of interpretability, bias, and overfitting are areas of ongoing research. The future holds promises of enhanced architectures, more efficient training methods, and the integration of neural networks with other advanced technologies like quantum computing.
Conclusion
In the intricate tapestry of Artificial Intelligence, neural networks weave a narrative of learning, adaptation, and unparalleled capabilities. This blog has laid the groundwork for understanding these powerful constructs, demystifying the core principles that drive AI applications and shaping the way we interact with intelligent systems.
---
"Unravelling the Mysteries of Neural Networks: A Beginner's Guide" provides a foundational understanding of neural networks, from their basic structure to real-world applications. Whether you're a newcomer to AI or seeking a refresher, this guide invites you into the captivating world of neural networks.
Comments